Author: Harish Seetamraju
A Deep Dive into the Casual Restaurant Industry’s Forecasting Solutions, and an Investigation of What’s in Their Future.
George Bernard Shaw, celebrated playwright, and Nobel Laureate, once said “there is no sincerer love than the love of food.” This persistent love for food coupled with the growing culture of eating out has steadily advanced over the last two decades. No matter the era, the two continue to power the growth of the food services industry. But there’s more on the plate for the food services industry than ever before. Along with serving food, restaurants and cafes have emerged as primary social hotspots. As a result of these factors, the sector and the opportunity therein continue to expand. The global food services market is worth USD 3.4 trillion (2018) and is expected to reach USD 4.2 trillion by 2024 at a CAGR of 3.6%. Close to a fourth of this rising figure is contributed by the US alone, where the market is divided between full service, limited service, and quick service establishments. The industry has grown a whopping 20-fold in the last half-century (from USD 43 billion in 1970 to USD 833 billion in 2018), with a million+ restaurants and 15 million+ employees today. Fast food occupies the lion’s share of the market owing to the convenience, cost, and speed of service it offers.
What does this ever-rising demand (~100 million orders served every day) mean for restaurant operations? Operating challenges such as increasing labor costs, rising labor turnover and excessive food wastage are a portion of the problem. Restaurateurs are also grappling with the demand for healthier and customized menu options while battling competition from low-cost ghost kitchens. The cost of goods sold along with the cost of labor makes up 60 to 70% of all operating costs incurred by a restaurant. This is why the old adage ‘every dollar saved is a dollar earned’ is ever-relevant for the sector and can help inform strategic decisions even today. A strategy focused purely on cost reduction might be risky, particularly if the restaurant loses sight of customer service or stock availability. But on the other hand, accurately anticipating demands and in response, aligning the inventory and labor can have a meaningful impact on costs, customer service, and ensure the often-perishable inventory isn’t booted to the wastebasket.
Looking beyond shareholder interests, food waste remains one of humanity’s greatest challenges. There’s a growing consensus that reducing food waste is key to our journey to meeting global food demands. It’s important, then, to note that there is still hope as data science attempts to solve the issue.
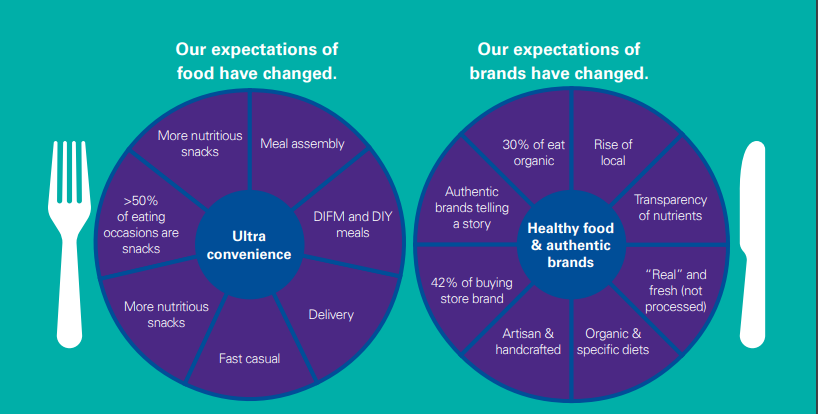
A Blueprint for Demand Forecasting
The simplest way to predict the future is by studying the past. As Mark Twain once explained, “History doesn’t repeat itself, but it often rhymes,” we know that there is much to decode from the patterns that have already presented themselves. Many restaurateurs have endeavored to do so, with varying degrees of sophistication, from simple spreadsheets to complex restaurant management applications that forecast future business outcomes. But while demand forecasting is an old puzzle, new solutions have now risen to make sense of the past. There is a need for stakeholders to evolve with the technological advances made in the field of computing and artificial intelligence to conquer the triple bottom line. Although many chain restaurants have central demand forecasting solutions in place, the store managers still manually adjust the forecast for notable discrepancies such as local road closures, adverse weather, or even a local event. Thus, the need of the hour is a solution that takes all the manual intervention and guesswork out of the equation by leveraging the learning ability of machines.
To that end, this article will look at the fundamental building blocks that form the core of such a solution. It will also focus on recent industry trends in 2020 and their relevant implications on demand planning.
1. Define the problem and establish the governing principles
The ‘purpose’ of the forecast will govern the design of the solution. Identifying the purpose will help with the selection of the best-suited algorithm and program implementation. For example, financial planning might need weekly sales forecasts aggregated at a geographical level, but labor scheduling needs projections for all labor drivers for each store by the hour. While the purpose may vary, some of the key considerations in this regard include:
● What should you forecast? Should you consider the number of items, the number of guests, or the money made in sales? Several items can be grouped if they drive the same labor.
● How far ahead should you forecast for? Inventory is typically ordered based on the most recent forecast, while labor may need to be scheduled two to three weeks in advance.
● How detailed should each forecast be? While a store manager may only need a daily item-level forecast to successfully order inventory, they would also want to see expected sales and guests for each day to be able to plan the level of labor required.
● How often do you need to generate forecasts?
● At what scale of operations will the forecasts be deployed? This will influence the scalability of the solution design and infrastructure.
● Are there downstream systems (either existing or in development) already in place that harness these forecasts for consumption at endpoints? Given that forecasts are only a means to an end, this step is crucial to be able to translate forecasts into business value while evaluating the solution.
Once the governing principles are in place, two factors determine the business value of the solution – comprehensive evaluation of demand drivers and choice of modeling algorithm.
2. Comprehensive evaluation of demand drivers
It’s imperative to evaluate the demand drivers holistically. This helps attribute every spike and dip to its cause while building a robust solution. A notable prerequisite is the availability of data for the past period at the required granularity. It’s not uncommon to use three to five years of time-series data to capture trends and seasonal influences.
Accounting for trend and seasonality in the to-be-forecasted time series is typically taken care of in solutions such as Facebook Prophet or Amazon Deep AR. It’s important to note that the time series should be made stationary when exploring more rudimentary methods such as Moving averages (MA) or Simple Exponential Smoothing (SES).
Additionally, internal factors that need evaluation include store operating hours, closures and remodels, price changes, new product launches, product and brand promotions. Every detail from happy hours to weekend buffets can influence demand. Including an on/off flag for a promotion versus engineering several features on what the promotion entails could give you significantly different reads.
External factors include holidays, national and local events, economic indicators (especially since this type of spending is discretionary), weather, and change in competition around the store within a particular radius. Every small element helps make the larger picture much clearer in a bid to demystify demand.
3. Choose a suitable algorithm that ticks all the boxes
There is a vast range of demand forecasting solutions in the industry including simple moving averages, exponential smoothing, ARIMA, libraries like Prophet, and even more advanced algorithms that use neural networks. Without dwelling on every detail pertaining to the methods, some factors that can help one decide which one is more suitable for a particular business are outlined below.
While accuracy is central to the whole exercise, production-grade solutions also need to consider scalability and maintainability depending on the scale of operations. Based on one’s business needs, explain-ability (for attributing changes in demand to a cause) could be important as well. For example, a marketing team might gain much more value if the model illustrates which promotions were more impactful.
In most cases, a trade-off might be necessary. Consider a chain restaurant with 5,000 stores and 200 unique products, collectively adding up to a million time-series. Parametric models fitted for each time series can reveal what caused the spike in demand for a particular week. But this could shoot up the maintenance cost. A neural network might bring down the maintenance costs by leveraging joint time series modeling along with the functionality to predict the demand for new products but at the cost of explainability.
Accuracy could be traded off as well depending on the use case. Many data scientists may go overboard when tuning the models, but the cost needs to be weighed against the incremental benefit. An improvement in forecast accuracy from 80% to 90% on the item forecasts could have a visible impact on the bottom line (in terms of reduced stock-outs). But every point improvement beyond that may not justify the cost incurred. The business may even define different thresholds for different time series. For example, fast-moving items could have a higher acceptance level vs long-tail items. To enable smart decisions for the most advantageous outcome, forecast accuracy needs to be translated to business KPIs based on the use case. This rule of thumb can guide decision-making when on the hunt for the right solution.
Demand Forecasting in 2020 and Beyond – A Future-ready Perspective
While the fundamentals of demand forecasting remain the same, there is a need to continuously evolve the solution to accommodate the ever-changing market dynamics and its unexpected shocks.
2020 will be known for its black swan event – COVID-19. The pandemic was not only a public health crisis but also an economic one, with governments across the world locking down all economic activity to contain the spread of the virus. As the crisis spread globally, the food industry was one of the worst-hit sectors. While many small businesses had to shut shop entirely, some of the larger chains were able to adapt to the situation, albeit slowly. Restaurateurs, those who can read into consumer behavior and the change in spending, may emerge stronger in the new normal. Advanced data science capabilities will become even more indispensable in navigating the post-Covid world. It’s a time that requires rigor in demand forecasting, one that allows for accuracy as well as adaptability. Some of the major considerations for forecasting in such an unprecedented time are outlined as follows.
● Porting to a forecasting solution that is sensitive to similar shocks and can adapt quickly may be hugely advantageous. Especially if the solution in use is not designed for it already.
● Given the uncertainty and multiple recovery paths, the forecasts should cover all possible futures. For the downstream decision-maker, a distribution of projections covering multiple possible scenarios, rather than a point forecast, would be more actionable.
● It could be fruitful to augment machine intelligence with that of humans. A provision to apply manual post model adjustments based on the most recent trends at the local level could prove really useful. These can also be captured as feedback into the model for future forecasts. The key here is being able to act on something the machine is not capturing, in an agile manner.
● Many restaurants are reducing their offerings and ingredients to deal with the disrupted supply chains. Tagging products with their attributes in the forecasting models can segment the time series into chunks that reveal similar and contrasting behaviors. For example, the disruption to schools and work have impacted breakfast sales. Drive-through sales have witnessed decent growth, capturing the fallout demand from dine-ins. Tagging of menu items and channel tagging of orders will enable the model to identify these patterns.
Despite the devastating loss, every disaster provides some opportunity. Amidst this crisis, food delivery has been a bright spot and has accelerated the adoption of digital channels for food order placements. The implications for demand forecasting are wide-ranging and have far-reaching impacts. There cannot be a one-size-fits-all model. Each channel will need independent projections given that the demand drivers might behave differently. For example, extreme weather might reduce dine-in demand but increase the demand for food delivery. Promotions of the delivery platforms themselves might drive digital sales as well. Meanwhile, the density of ghost kitchens in a particular radius needs to be accounted for when gauging competition. The intelligence from ordering platforms could also be fed into demand patterns for specific categories. In the long run, digital channels also ensure that customers leave a trail of their online footprint, which enables a deep dive into their preferences and purchase patterns for specific products in advance. Intelligence such as this makes forecasting foolproof and helps us help businesses protect themselves against catastrophes such as this.
Tiger Analytics develops custom-built demand forecasting solutions, tailored to the needs of each business and has delivered substantial value to our existing customers. As a result of our partnership, one of the largest fast-food chains in the US with 10,000+ stores was able to bring down their stock-outs by as much as 90%. Moreover, they were able to lower their stock holding cost by 20%. All of this was made possible with a custom-built forecasting solution.
References:
https://www.researchandmarkets.com/reports/4828753/food-service-market-global-industry-trends
https://restaurant.org/Downloads/PDFs/Research/SOI/2020-State-Of-The-Industry-Factbook.pdf
https://www.mordorintelligence.com/industry-reports/us-food-service-restaurant-market